Invited Talks
AAMAS 2017 is honored that the following colleagues will be giving invited talks at the conference:
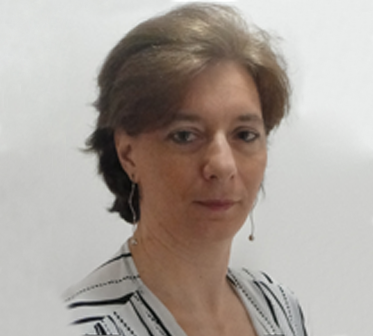
Ana Bazzan
Federal University of Rio Grande do Sul
Title: Trying to Fix Traffic: Past, Present, and Some Future Trends
ABSTRACT: According to the INRIX Global Traffic Scorecard, in 2016, an average driver in Los Angeles has spent 100 hours (approximately 4 days) in traffic jams. The economic impact of this is huge. Besides, also issues related to environment is non-negligible.
Many naive solutions tend to ignore the fundamental rule of traffic: building new roads just makes people drive more, i.e., more supply creates more demand. Given the continuous growth of the world urban population and the increasing needs related to mobility of people and goods, the demand is likely to grown anyway.
Lucky, there are not only bad news here: people do adapt to congestion. Therefore, it is important to understand how this adaptive process occurs, and how to seize the opportunity to develop or enhance agent-based tools as well as reinforcement learning methods in order to contribute to the effort of fixing traffic as much as possible. Among many others, one example here is the design of protocols and mechanisms underlying new traffic-related apps.
In this talk, I try to take a look at the present and future of this task. However, we should not throw away the past experience, despite new actors or players in this game, despite new paradigms that are starting to arise, and in spite of changes in preference by those actors (e.g., drop in car ownership among young people).
Thus, this talk reviews what we already know, as well as what we have already tried (for instance regarding the role of traffic control or regarding what can be learned from studying traffic assignment and route choice). Interesting questions here are: how can a microscopic, agent-based approach to modeling and simulation help decision-makers and traffic planners? How can new technologies, such as routing apps, microblogs, and context for prediction of patterns be incorporated to the past effort/agenda? How to most effectively use the internet of vehicles?
Finally, this talk summarizes some challenges and obstacles, such as the lack of testbeds, or even the lack of validation data.
SHORT BIO: Ana L. C. Bazzan holds a PhD degree from the University of Karlsruhe in Germany, and is a full professor at the Informatics Institute of the Federal University of Rio Grande do Sul (UFRGS) in Brazil. She has served as general co-chair of the AAMAS 2014 and is serving as one of the PC chairs of the PRIMA 2017 and one of the area chair of the IJCAI 2017 conference. She has served several times as member of the AAMAS (and other conferences) program committee (as PC member or senior PC member) and as an associated editor for: J. of Autonomous Agents and Multiagent Systems, Advances in Complex systems, and Mutiagent and Grid Systems. She is a member of the IFAAMAS board (2004-2008 and 2014-). She co-organized the Workshop on Synergies between Multiagent Systems, Machine Learning, and Complex Systems (TRI 2015), held together with IJCAI 2015, and the Workshop Agents in Traffic and Transportation (ATT) series. Her research interests include MAS, ABMS, machine learning, multiagent reinforcement learning, evolutionary game theory, swarm intelligence, and complex systems. Her work is mainly applied in domains related to traffic and transportation.
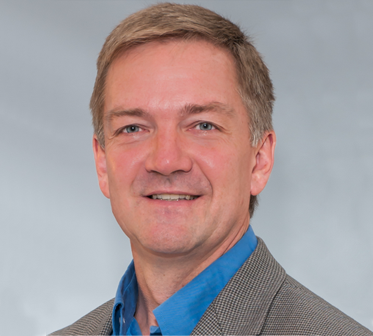
Jeff Schneider
Carnegie Mellon University, Uber ATG
Pittsburgh, PA
Title: Active Optimization and Self Driving Cars
ABSTRACT: Self driving cars hold the promise of making transportation safer and more efficient than ever before possible. They will also be among the most complex robotic systems ever fielded and thus require an unprecedented level of machine learning throughout to achieve their desired performance. These learners create an ever-changing environment for all algorithms operating in the system and optimizing their performance will become a perpetual activity rather than a one-off task.
I will present active optimization methods and their application in robotics applications, focusing on scaling up the dimensionality and managing multi-fidelity evaluations. I will summarize lessons learned and thoughts on future directions as these methods move into fielded systems. Finally, I will describe current efforts on self driving cars and give some views on the future of them.
SHORT BIO:
Dr. Jeff Schneider is the engineering lead for machine learning at Uber's Advanced Technologies Center. He is currently on leave from Carnegie Mellon University where he is a research professor in the School of Computer Science. He has 20 years experience developing, publishing, and applying machine learning algorithms in government, science, and industry. He has over 100 publications and regularly gives talks and tutorials on the subject.
Previously, Jeff was the co-founder and CEO of Schenley Park Research, a company dedicated to bringing machine learning to industry. Later, he developed a machine learning based CNS drug discovery system and commercialized it during two years as Psychogenics' Chief Informatics Officer. Through his research, commercial, and consulting efforts, he has worked with dozens of companies and government agencies around the world.
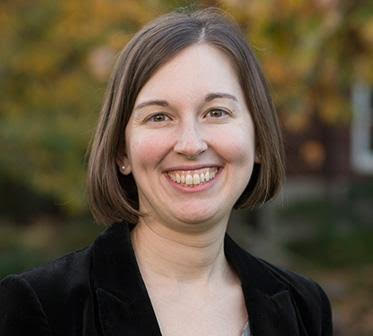
Julie Shah
Massachusetts Institute of Technology
Cambridge, MA
Title: Enhancing Human Capability with Intelligent Machine Teammates
ABSTRACT: Every team has top performers -- people who excel at working in a team to find the right solutions in complex, difficult situations. These top performers include nurses who run hospital floors, emergency response teams, air traffic controllers, and factory line supervisors. While they may outperform the most sophisticated optimization and scheduling algorithms, they cannot often tell us how they do it. Similarly, even when a machine can do the job better than most of us, it can’t explain how. In this talk I share recent work investigating effective ways to blend the unique decision-making strengths of humans and machines. I discuss the development of computational models that enable machines to efficiently infer the mental state of human teammates and thereby collaborate with people in richer, more flexible ways. Our studies demonstrate statistically significant improvements in people’s performance on military, healthcare and manufacturing tasks, when aided by intelligent machine teammates.
SHORT BIO: Julie Shah is an Associate Professor of Aeronautics and Astronautics at MIT and director of the Interactive Robotics Group, which aims to imagine the future of work by designing collaborative robot teammates that enhance human capability. As a current fellow of Harvard University's Radcliffe Institute for Advanced Study, she is expanding the use of human cognitive models for artificial intelligence. She has translated her work to manufacturing assembly lines, healthcare applications, transportation and defense. Before joining the faculty, she worked at Boeing Research and Technology on robotics applications for aerospace manufacturing. Prof. Shah has been recognized by the National Science Foundation with a Faculty Early Career Development (CAREER) award and by MIT Technology Review on its 35 Innovators Under 35 list. Her work on industrial human-robot collaboration was also in Technology Review’s 2013 list of 10 Breakthrough Technologies. She has received international recognition in the form of best paper awards and nominations from the ACM/IEEE International Conference on Human-Robot Interaction, the American Institute of Aeronautics and Astronautics, the Human Factors and Ergonomics Society, the International Conference on Automated Planning and Scheduling, and the International Symposium on Robotics. She earned degrees in aeronautics and astronautics and in autonomous systems from MIT.